
Using Optimisation and Machine Learning to Identify Optimum Store Locations, Numbers, and Formats




Optimisation – the key to solving complex network challenges
BearingPoint has been using analytics to support its supply chain projects for many years. Optimisation is a key tool, especially for network optimisation projects, but typically would be combined with other analytical methods.
We will present a short overview of the methodology to assess the future store network for a retailer, taking into account the changing behaviours of customers, with a blend of in-person and digital shopping.
Using client examples, we will demonstrate the mixture of machine learning (mostly clustering and determination of loss functions) and optimisation (for the actual network optimisation), to combine the statistical elements of the question with the prescriptive outputs generated through the optimisation model.
Finally, we will highlight some key outputs from a recent study as well as some lessons learned.
Emile Naus, Partner, BearingPoint
Emile is the UK Partner for Operations. He has 30 years’ experience in designing, building and running supply chains. He was previously Head of Logistics Strategy for Marks and Spencer and Long Term Planning Manager for Tesco.
Paul Derbyshire, Senior Technical Advisor, BearingPoint
Paul is a Senior Technical Advisor within the Operations team at BearingPoint UK. Paul has over 20 years’ experience in supply chain analytics and modelling. Prior to BearingPoint, Paul worked as consultant, implementing planning and scheduling optimisation solutions in the Process industries.
Customers
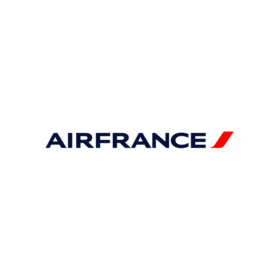

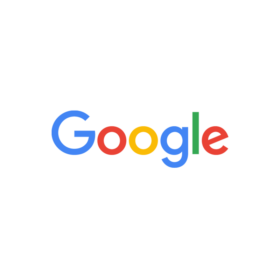
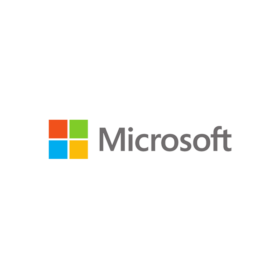
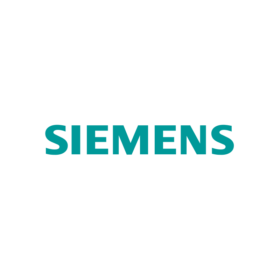
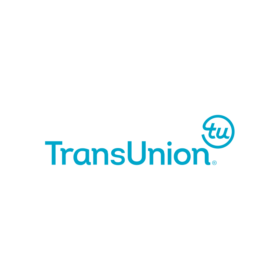
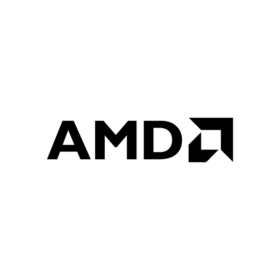
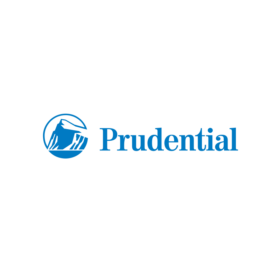